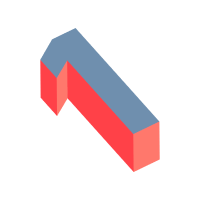
Initiate
- AML experts from TMNL, banks and public parties discuss money laundering risks and patterns that TMNL could analyse.
- TMNL coordinates its proposal for possible models with national priorities in the fight against money laundering, addressing money laundering in a way that banks in isolation cannot.
- Based on inputs from the wider stakeholder field, TMNL proposes developing a specific model (e.g. on underground banking) as part of its model roadmap.
- On approval by the banks, the actual building starts.